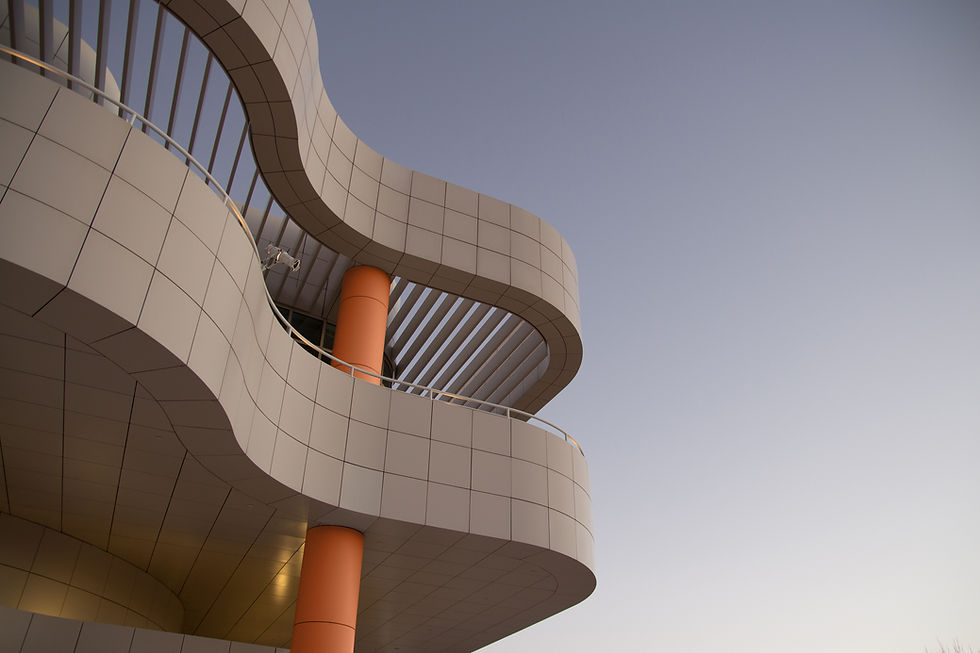
Computer Vision and GenAI: Revolutionizing Industries through Visual Intelligence
Oct 20, 2024
2 min read
0
12
0
From analyzing images of vast landscapes to generating synthetic digital twins, computer vision—powered by Generative AI (GenAI)—is transforming industries such as agriculture, manufacturing, and healthcare. As AI-driven visual intelligence advances, it is significantly accelerating productivity, efficiency, and decision-making processes across these sectors. However, the journey toward fully integrating GenAI into computer vision is not without its challenges, and key issues such as data interpretability, processing power, and privacy concerns are becoming central to the conversation.
In a recent panel discussion, experts explored these challenges and delved into the emerging trends in the field of GenAI for computer vision. A major barrier identified was the enormous volume of data and the processing power required to efficiently train and deploy AI models. Computer vision systems rely on vast amounts of visual data—satellite images, medical scans, industrial footage—often at a scale that is difficult to manage. For GenAI to become more widely applicable, there needs to be significant advances in data processing capabilities, as well as the optimization of models to handle large datasets more effectively.
Another key issue is data governance and privacy, particularly in healthcare. As computer vision applications in healthcare grow—from medical imaging to real-time diagnostics—there is an increasing need for stringent protocols around patient data security. Protecting sensitive health information, while still leveraging AI for deeper insights, is a complex balancing act. Implementing robust frameworks for data privacy and regulatory compliance is critical for the safe and ethical adoption of AI technologies in this space.
Despite these hurdles, one of the most exciting trends in computer vision is the rise of multimodal learning—the integration of visual data with other forms of data, such as text, audio, or sensor data. Multimodal models are designed to analyze and synthesize information from multiple domains, providing richer insights and enabling more comprehensive data analysis. For example, in agriculture, combining visual data from drones with weather data and soil sensors can generate highly accurate predictions about crop health and yield. In healthcare, integrating medical images with patient records and clinical notes can create more personalized treatment plans.
The future of computer vision, especially when powered by GenAI, lies in this multimodal fusion of information. As AI systems become better at interpreting diverse datasets, they will unlock new possibilities for innovation across industries. Multimodal models promise to deliver deeper insights, drive smarter decision-making, and improve efficiency in ways that are more holistic and context-aware.
In conclusion, while there are still challenges to overcome—such as scaling processing power, ensuring data privacy, and improving interpretability—GenAI is poised to revolutionize computer vision. By integrating visual data with other data types through multimodal learning, we are moving toward a future where AI can offer not just specialized insights, but a comprehensive understanding of complex systems across industries. This transformation will continue to shape the landscape of computer vision, bringing AI-powered advancements to the forefront of innovation.